Invited Talk by Danielle Pace
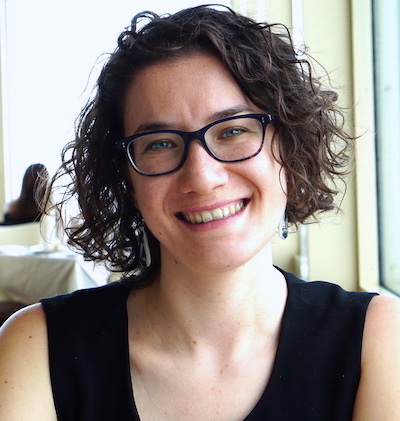
|
Danielle Pace
Department of Computer Science at the Massachusetts Institute of Technology
- Date: Friday, May 24th, 2019
- Time: 11 AM
- Location: MI 03.13.010
|
Whole heart segmentation in congenital heart disease by learning iterative segmentation
This work addresses the need for whole heart segmentation to individually label the cardiac chambers and great vessels for patients with congenital heart disease. Our main challenges are extreme anatomical variability and very limited training data. We demonstrate an iterative segmentation method, implemented as a recurrent neural network (RNN), in which a user provides a single landmark per structure, and a segmentation is evolved over multiple steps until reaching a stopping point that can be user-defined or automatically determined. The model grows segmentations in a predictable way that is defined during training. We show that a loss function that evaluates the entire sequence of output segmentations can be optimized using training images alongside input-output pairs of partial segmentations. Our experiments demonstrate that, compared to conventional models that segment an image in one step, the iterative segmentation offers better generalization to patients with the most severe heart malformations.
Danielle Pace is a Ph.D. Candidate in Computer Science at the Massachusetts Institute of Technology, advised by Dr. Polina Golland. Her current work is in machine learning for image segmentation, specifically for challenging clinical applications involving wide anatomical variability and little training data. Previously, Danielle was a Research and Development Engineer at Kitware, Inc., where she worked on problems in image registration. She received a Master's in Biomedical Engineering from The University of Western Ontario, where she developed techniques for ultrasound reconstruction applied to image-guided cardiac interventions. Her Bachelor's is in Biomedical Computing from Queen's University.